New NIST standards disallow the use of VoIP-based phone numbers for SMS two-factor authentication. Argh, if I can’t use my Google Voice number, how can I receive SMS codes overseas without paying exorbitant telco roaming charges? I don’t consider a mobile-OS 2FA app to be a reliable substitute.
Author: Dan Maas
Looking for a Database Console
I’m looking for any existing tools to manage a set of several MySQL or Postgres databases. Something like the Amazon RDS console, but with the ability to dump and restore data to plain SQL files instead of just filesystem snapshots.
Terraform Tips
Terraform is a wonderful declarative system for setting up cloud resources – sort of a vendor-neutral AWS CloudFormation with nicer syntax.
See https://charity.wtf/tag/terraform/ for a gold mine of Terraform information.
I’m writing this because I just experienced the “joy” of losing my Terraform state file for the first time, which means I need to go and delete 53 AWS resources by hand!
Database Horror Stories
If databases are an essential part of your work, and you’d like some nightmare stories to keep you awake at night, check out https://aphyr.com. The author devastates the consistency, availability, and partition tolerance of supposedly “robust” modern databases.
Warcraft: The Movie Reactions
This film is just what it needed to be: first, a fantastic piece of WoW-gamer fan service, and second, an adequate if uneven opening to what will hopefully become a solid fantasy film franchise.
I expect Warcraft to receive poor reviews from mainstream film critics who aren’t familiar with the game world. It doesn’t function well as a stand-alone story compared to past fantasy epics. But, I think the critics will be surprised by its commercial success. I expect the follow-up sequels to feature more solid direction now that the franchise has a grounding in Hollywood – just as the Lord of the Rings films got stronger as the trilogy developed.
ILM did an amazing job on the CGI characters – most of whom acted more believably than the humans! I loved the subtle facial expressions when the orcs teased each other.
The violence seemed overdone, but I imagine having so many bloody war scenes was a requirement to ensure success in non-English markets in case the subtler drama failed to connect.
Some of the human roles felt mis-cast and performed (or were directed?) half-heartedly. For example, I felt he quiet scene between Medivh and Garona where they discuss lost families fell flat.
Seeing the WoW world in high-resolution film was amazing. I particularly loved seeing Karazhan, Dalaran, and what looked like part of the Nexus, after spending so much time in those places in game. Stormwind was perfect down to the classic circular candelabras. Playing mostly on the Horde side myself, I can’t wait to see more of the orc, Tauren, and Blood Elf worlds – though the Blood Elves looked terribly silly in this film, and I hope they get re-designed for any sequels.
Lastly, I very much enjoyed the subtler WoW references, like Khadgar explaining how Polymorph works “for 60 seconds, and only on the weak-minded,” and Lothar’s response that his last visit to Karazhan was about six years ago – yeah, me too buddy!
Susskind’s Lectures on Physics
I highly recommend the extensive series of physics lectures by Prof. Leonard Susskind now available on YouTube! (complete playlist link)
Susskind does a great job explaining advanced physics without the overwhelming technical jargon that makes Wikipedia pages and papers on these topics incomprehensible to me.
With some background in undergraduate physics I was able to start with the “New Revolutions in Particle Physics” sequence and, for the first time, begin to understand what is happening in quantum field theory.
Anyone with a computer graphics background will find Lectures 3 and 4 of the Standard Model series familiar, in which Susskind derives some properties and relationships of rotation matrices. This leads neatly into group theory and some understanding of what the legendary “U(1)xSU(2)xSU(3)” actually means.
How AlphaGo Works
Today Google DeepMind announced a neural-network AI for the game Go, AlphaGo, that rivals the strength of human professional players. The paper by David Silver et al describes AlphaGo in detail. Their technique is surprisingly simple yet very powerful. Here is my attempt to explain how the system works, for readers not familiar with the technical jargon used in the paper.
中文译版(Credit: 数据分析网):http://www.afenxi.com/post/8713
Deep Learning
“Deep learning” refers to multi-layered artificial neural networks and the methods used to train them. A neural network layer is just a large matrix of numbers that takes a set of numbers as input, weighs them through a nonlinear activation function, and produces another set of numbers as output. This is intended to mimic the function of neurons in a biological brain. With the right numbers in that matrix, and several of these layers hooked together in a chain, a neural network “brain” can perform accurate and sophisticated processing, like recognizing patterns or labeling photos.
Neural networks were invented decades ago, and mostly lay in obscurity until just recently. This is because they require extensive “training” to discover good values for the numbers in those matrices. The minimum amount of training to get good results exceeded the computer power and data sizes available to early neural network reserachers. But in the last few years, teams with access to large-scale resources have rediscovered neural networks, which are now possible to train effectively using “Big Data” techniques.
Two Brains
AlphaGo is built using two different neural-network “brains” that cooperate to choose its moves. These brains are multi-layer neural networks almost identical in structure to the ones used for classifying pictures for image search engines like Google Image Search. They start with several hierarchical layers of 2D filters that process a Go board position just like an image-classifying network processes an image. Roughly speaking, these first filters identify patterns and shapes. After this filtering, 13 fully-connected neural network layers produce judgments about the position they see. Roughly, these layers perform classification or logical reasoning.
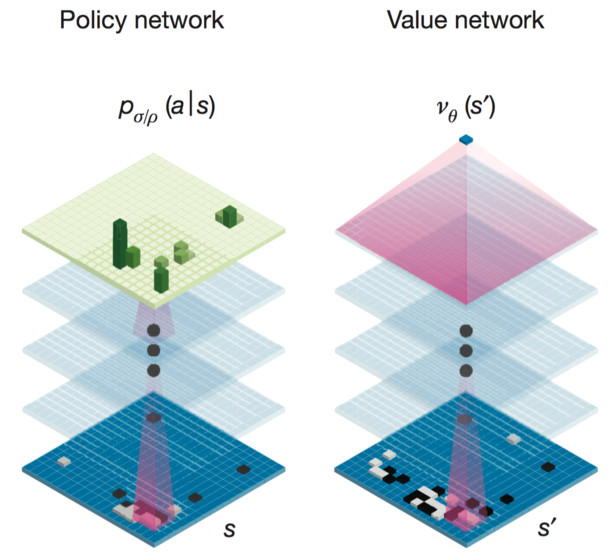
The networks are trained by repeatedly checking their results and feeding back corrections that adjust the numbers to make the network perform better. This process has a large element of randomness, so it’s impossible to know exactly how the network does its “thinking”, only that it tends to improve after more training.
Brain #1: The Move Picker
AlphaGo’s first neural-network brain, which the paper refers to as the “supervised-learning (SL) policy network”, looks at a board position and attempts to decide the best next move to make. Actually, it estimates the probability that each legal next move is the best, where its top guess is the one with the highest probability. You can think of this as a “move-picking” brain.
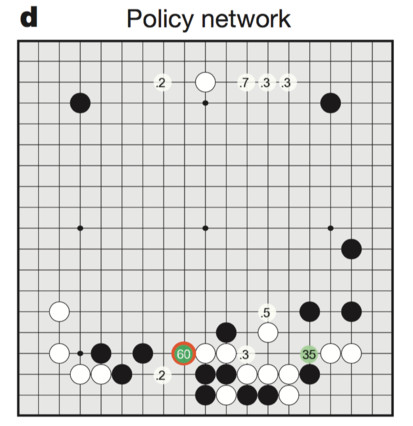
Silver’s team trained this brain on millions of examples of moves made by strong human players on KGS. It’s the most human-like part of AlphaGo, in that its goal is only to replicate the move choices of strong human players. It expressly does not care about winning games, only picking the same next move that a strong human player would pick. AlphaGo’s move-picker correctly matches strong humans about 57% of the time. (mismatches aren’t necessarily a mistake – it might be the human who made the wrong move!).
The Move Picker, Stronger
The full AlphaGo system actually needs two additional versions of the basic move-picking brain. One version, the “reinforced-learning (RL) policy network”, is a refined version that is trained more intensively using millions of additional simulated games. Think of this as the “stronger” move picker. In contrast to the basic training described above, which only teaches the network to mimic a single human move, the advanced training plays out each simulated game to the end in order to teach the network which next moves are likely to lead to winning the game. Silver’s team synthesized millions of training games by playing the stronger move picker against previous editions of itself from earlier training iterations.
The strong move picker alone is already a formidable Go player, somewhere in the amateur low-dan range, on par with the strongest pre-existing Go AIs. It’s important to note that this move picker does no “reading” at all. It simply scrutinizes a single board position and comes up with one move based on its analysis of that position. It does not attempt to simulate any future moves. This shows the surprising power of simple deep neural-network techniques.
The Move Picker, Faster
The AlphaGo team did not stop here, of course. In a moment I will describe how they added reading ability to the AI. In order for reading to work though, they needed a faster version of the move-picking brain. The stronger version takes too long to produce an answer – it’s fast enough to produce one good move, but reading calculations need to check thousands of possible moves before making a decision.
Silver’s team built a simplified move picker to create a “fast reading” version, which they call the “rollout network”. The simplified version doesn’t look at the entire 19×19 board, but instead only looks at a smaller window around the opponent’s previous move and the new move it’s considering. Removing part of the move-picker’s brain in this way lowers its strength, but the leaner version computes about 1,000 times faster, making it suitable for reading calculations.
Brain #2: The Position Evaluator
AlphaGo’s second brain answers a different question than the move picker. Instead of guessing a specific next move, it estimates the chance of each player winning the game, given a board position. This “position evaluator”, which the paper calls the “value network”, complements the move picker by providing an overall positional judgment. This judgment is only approximate, but it’s useful for speeding up reading. By classifying potential future positions as “good” or “bad”, AlphaGo can decide whether or not to read more deeply along a particular variation. If the position evaluator says a particular variation looks bad, then the AI can skip reading any more moves along that line of play.
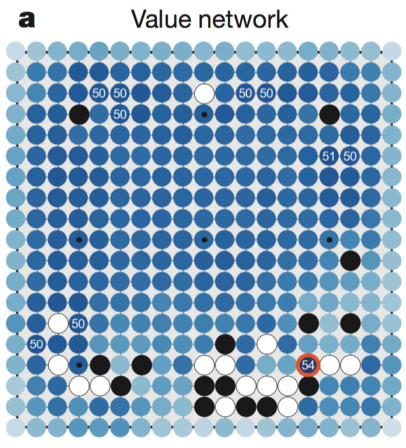
The position evaluator is trained on millions of example board positions. Silver’s team created these positions by carefully selecting random samples from simulated games between two copies of AlphaGo’s strong move-picker. It’s important to note that the AI move-picker was invaluable in constructing a sufficiently large data set to train the position evaluator. The move-picker allowed the team to simulate many possible forward continuations from any given board position to guess the approximate winning chances of each player. There probably aren’t enough human game records available out there to accomplish this kind of training.
Adding Reading
With all three versions of the move-picking brain, plus the position-evaluation brain, AlphaGo is now equipped to read sequences of future moves effectively. Reading is accomplished with the same Monte Carlo Tree Search (MCTS) algorithm used by most state-of-the-art Go AIs. But AlphaGo out-smarts other AIs by making more intelligent guesses about which variations to explore, and how deeply to explore them.
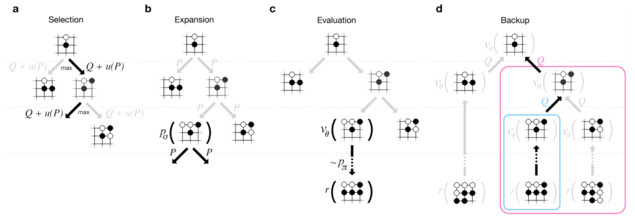
With infinite computer power, MCTS could theoretically compute optimal moves by exploring every possible continuation of a game. However, because the search space of future moves of a Go game is so large (greater than the number of particles in the known universe), no practical AI can hope to explore every possible variation. Getting MCTS to work in practice depends on how good the AI is at identifying promising variations, so that it can skip exploring bad ones.
Silver’s team equipped AlphaGo with a modular MCTS system. This framework allows the designers to “plug in” different functions for evaluating a variation. The full-power AlphaGo system uses all the “brains” in the following way:
1. From the current board position, choose a few possible next moves. For this they use the “basic” move-picking brain. (they tried using the “stronger” version in this step, but it actually made AlphaGo weaker, because it didn’t offer the MCTS system a broad enough choice of variations to explore. It focused too much on one “obviously best” move instead of reading several alternatives that might reveal themselves to be better later in the game).
2. For each possible next move, evaluate the quality of that move in one of two ways: either use the position evaluator on the board state after that move, or run a deeper Monte Carlo simulation (called a “rollout”) that reads into the future after that move, using the fast-reading move picker to speed up the search. These two methods produce independent guesses about the quality of the move. AlphaGo uses a single parameter, a “mixing coefficient”, to weigh these guesses against each other. Full-power AlphaGo uses a 50/50 mix, judging the quality equally using the position evaluator and the simulated rollouts.
The paper includes a neat graph that shows how AlphaGo’s strength varies as they enable or disable the use of the pluggined-in brains and simulations in the above steps. Using only one individual brain, AlphaGo is about as strong as the best computer Go AIs, but with all of them combined, it’s possibly achieved the level of a professional human player.
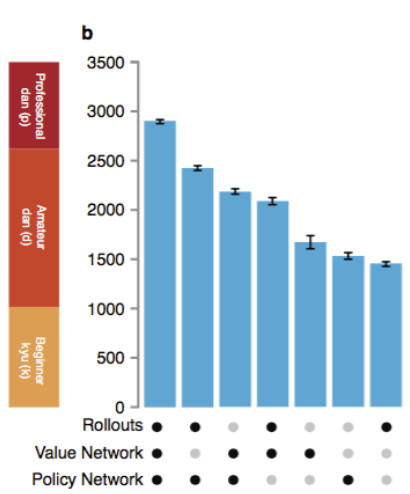
The paper goes into detail about some fancy engineering the team did to speed up MCTS by distributing the calculations across a network of computers, but this doesn’t change the fundamental algorithm. There is also an appendix with mathematical justifications for each step. Some parts of the algorithm are exact (in the asymptotic limit of infinite computer power) while some are only approximations. In practical terms, AlphaGo will certainly get stronger with access to greater computer power, but the rate of improvement per additional unit of computing time will slow down as it gets stronger.
Strengths and Weaknesses
Note: I’m only a weak amateur Go player so take my analysis with some skepticism.
I believe AlphaGo will be tremendously strong at small-scale tactics. It knows how to find the “best of” human moves played for many positions and patterns, so it likely won’t make any obvious blunders in a given tactical situation.
However, AlphaGo might have a weakness in “whole-board” judgment. It looks at board positions using a pyramid of 5×5 filters, which suggests that it might have trouble integrating tactical “parts” into a strategic “whole”, in the same way that image-classifying neural networks sometimes get confused by images that contain parts of one thing and parts of another. An example of this might be a game where a joseki in one corner creates a wall or a ladder-breaker that significantly changes the value of the position in a different corner.
Like other MCTS-based AIs, AlphaGo could have trouble judging positions that need very deep reading to resolve a big risk, like long ko seqeuences where the life and death of a large group is at stake.
AlphaGo could also be confused by deliberate attempts to deviate from a normal-looking game, like a tengen opening or other rare joseki, since much of its training is based on human game records.
I look forward to seeing the result of the upcoming match between AlphaGo and top human player Lee Sedol 9p! My prediction: if Lee plays “straight”, as if playing against another human professional, he will probably lose, but he might win if he can force AlphaGo into an unfamiliar strategic situation.
NewSQL
The current high-availability options for file sharing and SQL services seem shockingly primitive compared to what’s now available for modern web APIs and key/value stores like Amazon S3. “State of the art” servers like Owncloud are often in the Stone Age when it comes to having single points of failure. For example, Owncloud points to GlusterFS as a way of making its shared filesystem robust against a server failure, but if you read the GFS docs carefully, it looks like recovering from a failure is a seriously involved process that requires manually logging in (!) to fix things and get out of “degraded mode”.
Come on, it’s 2015! Individual server instances must be treated as disposable minions that can come and go at will. S3 doesn’t just “go down” when a single machine fails somewhere. It might glitch for a few seconds or require retrying a request, but that’s about it. There’s no “degraded mode”, just slightly more or less redundancy at any given time.
Even the stolid SQL databases like MySQL and Postgres offer very limited high-availability options (without exotic third-party plugins), basically consisting of a pair of duplicate servers where one can fail over to the other. The fail-over process is delicate; if something fouls up along the way, you could be left off-line for hours to resynchronize tables or rebuild them from backups. Surely this is not robust enough to handle something like a username/password database for Facebook.
After dabbling with MongoDB and looking at etcd for a while, I’ve begun to take for granted the notion of a self-healing cluster of individually unreliable server instances. When is this approach going to become an easy-to-use part of off-the-shelf databases? Standardizing this form of high-availability is important because, while you could write your own reliability layer on top of a set of unreliable servers, doing so is going to be labor-intensive to develop and difficult to maintain.
It turns out a lot of activity is taking place in this area now, and there’s even a name for the trend: “NewSQL”. The Usenix talk linked below is a good high-level overview of why and how databases are evolving in this direction:
Simplifying Asynchronous Code in Twisted
Twisted’s “Deferred” system for performing asynchronous operations is powerful and flexible, but very hard to learn. I’m not sure my mental model of how Deferreds work is fully correct even after several years of using them.
Recently I discovered there’s a far simpler way to drive asynchronous operations in Twisted: use the @defer.inlineCallbacks decorator, where you wait on the asynchronous result as if it were coming from a Python generator. Why didn’t I see this option three years ago?
The old “traditional” manual callback style:
def print_file(file_): d = async_print_file(file_) d.addCallback(on_success) d.addErrback(on_failure) def get_file(): d = async_get_file() d.addCallback(print_file) d.addErrback(on_failure)
where you end up creating awkward little classes to maintain the state you need from step to step in the chain of operations, and awkwardly hooking up argument lists with functools.partial etc.
The new generator-coroutine style is far more concise:
@defer.inlineCallbacks def get_and_print_file(): file = yield async_get_file() result = yield print_file()
The “yield from” expression suspends execution until the async operation completes. Errors are handled by placing try/except blocks in reasonable places.
Getting the details right
Two complications generally appear with simple-looking asynchronous code: error handling and management of in-flight requests. The inlineCallbacks system handles errors cleanly simply by throwing an exception from the “yield” when the call fails. It does not, however, offer any way to monitor the status of in-flight requests (e.g. to prevent an unbounded pile-up of requests to a failed server that is timing out). That still must be done manually where necessary.
The OpenXcom Engine
SupSuper’s open-source reimplementation of the original XCOM game engine, OpenXcom, is nearly complete! Check out the code at https://github.com/SupSuper/OpenXcom. Some notes for reading:
- There’s a simple build process with few external dependencies (just SDL and YAML).
- Several “make”-like systems are implemented in parallel. You can compare and contrast how CMake/autotools/XCode/MSVC look for the same code.
- The engine is somewhat modularized:
- “Basescape” for the base management GUI
- “Geoscape” for the world map
- “Battlescape” for tactical combat
- However, the headers aren’t organized clearly and there are some cross-includes between the different modules.
- Game data like item/tech states are parsed from YAML and accessed by a “Ruleset” library.
- The “Savegame” system is misleadingly named; it’s actually the container for all persistent player state.
- There are beautifully concise (1-page) implementations of A* and Dijkstra’s algorithm in Pathfinding.cpp
- Cringe at memory-management horrors like:
Craft::~Craft() { for (std::vector<CraftWeapon*>::iterator i = _weapons.begin(); i != _weapons.end(); ++i) { delete *i; } delete _items; for (std::vector<Vehicle*>::iterator i = _vehicles.begin(); i != _vehicles.end(); ++i) { delete *i; } }
(which translates to zero lines of code in modern RAAI C++)